AI (ML) has arisen as an extraordinary innovation that is reshaping enterprises and upgrading day to day existence. It permits PCs to gain from information and further develop execution without unequivocal programming, empowering applications as different as customized proposals, extortion location, and independent vehicles. This article investigates the basics of AI, its applications, center parts, and the difficulties and moral contemplations it presents. Furthermore, we incorporate an examination table of AI methods and a talk style table with featured discussions for speedy reference.
Understanding Machine Learning
AI is a subset of man-made consciousness (simulated intelligence) that spotlights on creating calculations fit for perceiving examples and pursuing choices in light of information. In contrast to conventional programming, where express directions are coded, ML models use information to adjust and learn over the long run, working on their precision and productivity.
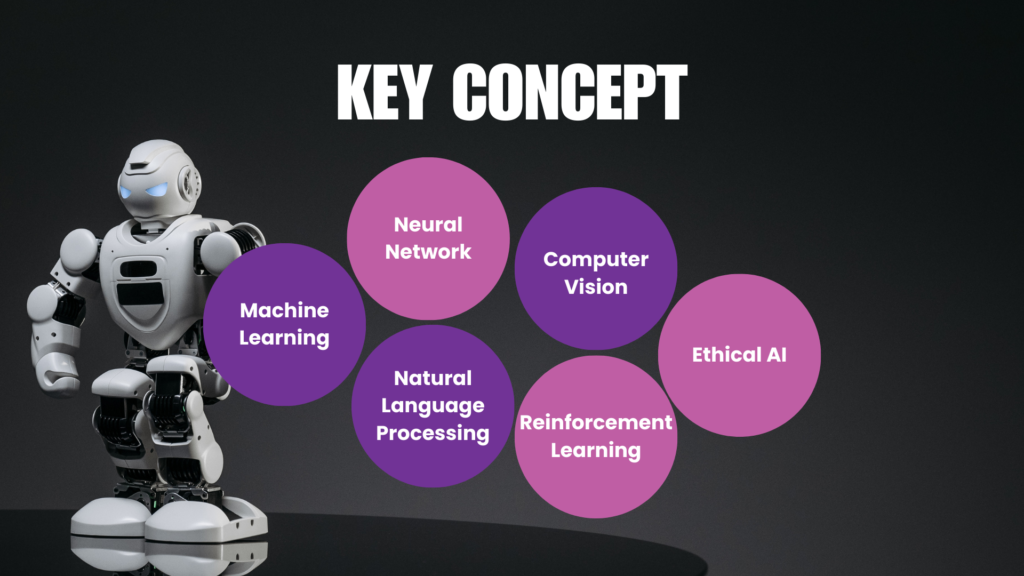
At its center, ML includes three vital sorts of learning:
- Managed Learning: Includes preparing a model on named information, where input-yield matches are given. Models incorporate spam email recognition and foreseeing house costs.
- Solo Learning: Works with unlabeled information to distinguish examples or groupings. Models incorporate client division and abnormality identification.
- Support Learning: Spotlights on independent direction by gaining from cooperations with a climate to boost a prize sign. Applications incorporate advanced mechanics and game playing.
Applications of Machine Learning Across Industries
Revolutionizing Healthcare with Precision
AI is fundamentally propelling medical care by empowering early illness location, customized therapies, and productive diagnostics. For example, ML calculations examine clinical pictures to distinguish anomalies like growths, while prescient models expect patient results, further developing consideration.
Enhancing Customer Experience in Retail
Retailers influence ML to propose customized proposals in view of shopper conduct. Calculations break down buy history, perusing examples, and inclinations to tailor ideas, streamlining client commitment and fulfillment.
Streamlining Financial Services
Monetary organizations use ML for extortion discovery, risk evaluation, and algorithmic exchanging. AI models recognize surprising examples in exchanges, hailing likely extortion continuously while upgrading dynamic in loaning and speculations.
Driving Innovation in Autonomous Systems
Self-driving vehicles, robots, and mechanical technology depend intensely on ML to handle sensor information, perceive items, and pursue split-subsequent options. This empowers robotization in transportation, agribusiness, and assembling, changing these areas.
Core Components of Machine Learning Systems
Understanding the structure blocks of AI is fundamental to valuing its capacities.
Data: The Foundation of ML
Great information is the foundation of any AI project. It should be significant, different, and liberated from inclination to guarantee precise model preparation. Information preprocessing, like cleaning and standardization, is a basic step.
Algorithms: The Brain of ML
AI calculations decide how information is handled and investigated. Normal calculations include:
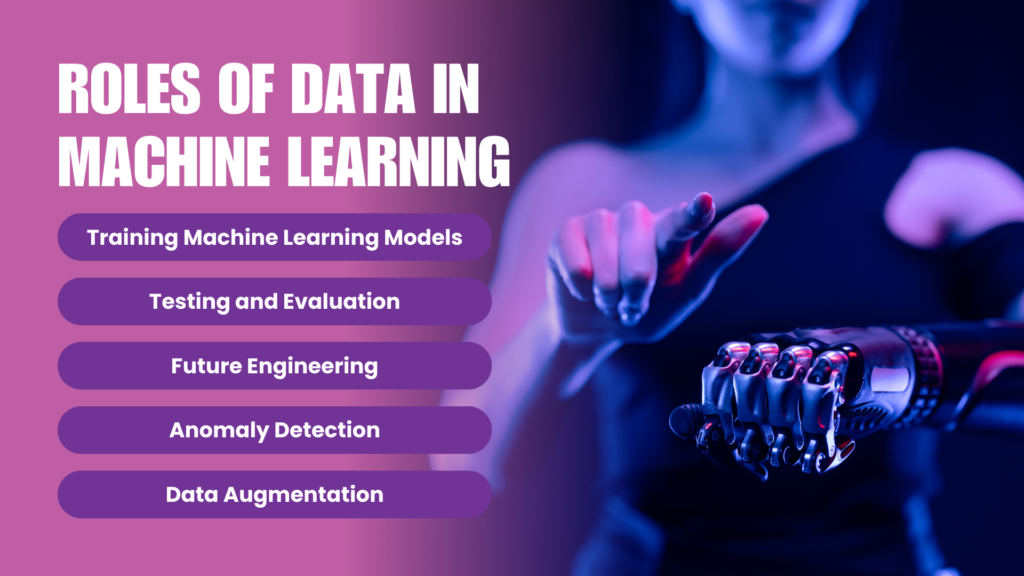
- Linear Regression: Used for predictive modeling.
- Decision Trees: Ideal for classification tasks.
- Neural Networks: The backbone of deep learning.
Training: Teaching the Model
During preparing, calculations learn designs by handling a dataset. This includes changing boundaries to limit mistake. Strategies, for example, cross-approval guarantee models sum up well to concealed information.
Evaluation: Measuring Performance
Measurements like exactness, accuracy, review, and F1-score assess the adequacy of ML models. These estimations help decide whether a model is prepared for sending.
Comparison Table: Machine Learning Techniques
The accompanying table thinks about key AI strategies in light of their attributes, benefits, and difficulties:
Technique | Characteristics | Advantages | Challenges |
---|---|---|---|
Supervised Learning | Labeled data required | Accurate for specific tasks | Requires large labeled datasets |
Unsupervised Learning | Works with unlabeled data | Discovers hidden patterns | Difficult to validate results |
Reinforcement Learning | Environment-based learning | Effective in dynamic environments | Requires extensive simulation |
Deep Learning | Multi-layered neural networks | Handles complex tasks like vision | Computationally expensive |
Ethical Considerations in Machine Learning
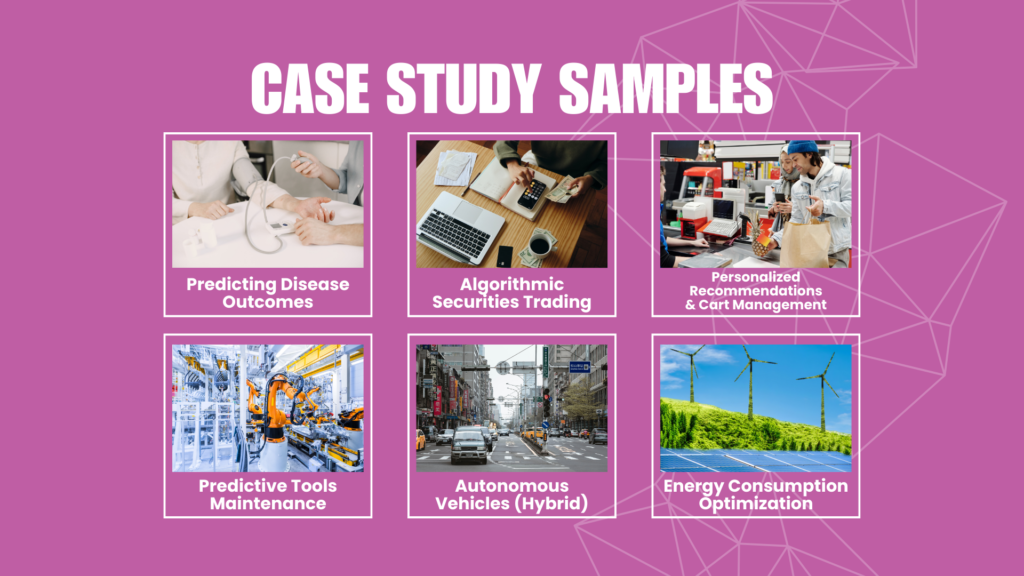
While ML offers gigantic potential, it likewise raises moral and cultural worries that should be tended to.
Ensuring Fairness and Reducing Bias
AI models can coincidentally propagate predispositions in the information they are prepared on. For example, one-sided employing calculations might lean toward specific socioeconomics. Guaranteeing reasonableness requires different preparation datasets and cautious observing.
Protecting Privacy and Data Security
ML frameworks frequently depend on huge datasets, including delicate individual data. Guaranteeing information security and following guidelines like GDPR is basic to keeping up with trust and safeguarding clients’ protection.
Managing Job Displacement
Mechanization driven by AI can prompt work removal in specific areas. Proactive labor force advancement and retraining programs are fundamental to moderate these impacts.
Chat Table: Key Notes on Machine Learning
Topic | Details |
---|---|
Types of Learning | Supervised, unsupervised, reinforcement |
Key Applications | Healthcare, retail, finance, autonomous systems |
Common Algorithms | Linear regression, decision trees, neural networks |
Challenges | Data quality, model bias, computational complexity |
Ethical Concerns | Fairness, privacy, job displacement |
Evaluation Metrics | Accuracy, precision, recall, F1-score |
The Future of Machine Learning
AI is developing quickly, with advancements promising significantly more noteworthy effect. Here are a few arising patterns:
Federated Learning: Enhancing Privacy
Combined gaining empowers ML models to gain from decentralized information sources without sharing delicate data. This approach upgrades protection and information security, especially in medical services and money.
Explainable AI: Improving Transparency
Reasonable simulated intelligence centers around making ML models interpretable, assisting clients with figuring out choices. This is especially significant in controlled businesses like money and medical care.
Edge Computing: Enabling Real-Time Processing
Edge figuring incorporates ML abilities straightforwardly into gadgets, empowering continuous direction. This is pivotal for applications like independent vehicles and IoT gadgets.
Conclusion
AI is changing ventures, engaging organizations, and working on day to day existence. Its capacity to dissect complex information, adjust to new data, and convey significant experiences makes it a foundation of current innovation. By grasping its center standards, tending to moral worries, and embracing future developments, we can bridle the maximum capacity of AI for a more brilliant, more fair future.
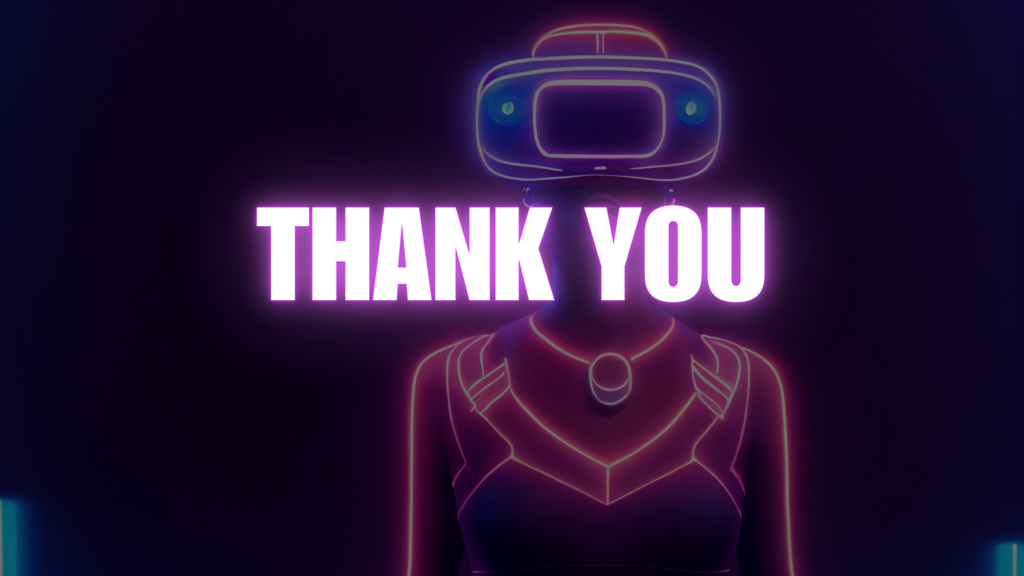